University of Sheffield Projects
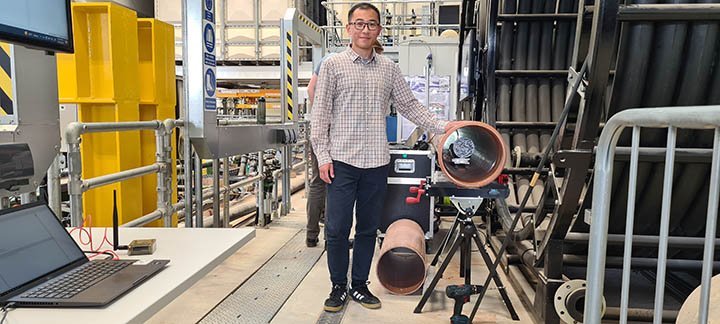
Autumn 2025 applications
If you want to know more about a project, please contact the named supervisors. You can also suggest your own project, but in 2025 most projects will be the ones funded by our partners.
Sh.24.1 PhD in Acoustics of Composite Fibres with Don & Low Ltd
- Project type: Industry-driven
- Supervisors: Kirill Horoshenkov and Anton Krynkin, University of Sheffield
- Project Partner: Don & Low Ltd
This is an exciting opportunity to carry out a world-class programme of research supported by a leading manufacturer of technical textiles products for applications in construction, geotextiles medical, Filtration and automotive markets. This research will be focused on the development of innovative new products with unique acoustics properties that can widen considerably the range of sectors and applications where these products can be applied. The student will be provided with a substantial financial support to travel extensively in the UK and overseas to visit potential partners, production sites and to present the results of their work at high-profile international events. The student will also receive extensive training in acoustics, machine learning, material science, communication skills, related legislation, outreach and communication skills. Furthermore, the student will gain valuable product development experience in one of Europe’s leading technical textiles manufacturers.
Specifically, the work will involve theoretical and numerical modelling, laboratory manufacturing, experiments and production trials of nonwoven textile laminates with the intent to obtained materials with optimised acoustic absorption and transmission making these materials highly attractive for a wide range of noise control applications. A key research challenge is to develop a good understanding of the relation between the textile production process, material microstructure and resultant acoustical and other useful material properties, e.g. filtration efficiency, fluid permeability and long-term stability. The development of advanced models for these materials would allow a parametric approach to perfect the material microstructure, inform and to modify if required the material manufacturing process to achieve the design performance. The developed models will also allow the industry partner to speed up the material development process in terms of its reproducibility, performance and cost.
The research would suit graduates in material science, chemistry, physics or related area of engineering. Experience in manufacturing and design will be useful. You will be based in the School of Mechanical, Aerospace and civil Engineering at the University of Sheffield.
Sh.24.2 PhD in Acoustic Performance, Analysis and Design of Steel Composites
- Project type: Industry-driven
- Supervisors: Anton Krynkin and Hassan Ghadbeigi, University of Sheffield
- Project Partner: Hadley Group, producer of cold rolled steel sections and allied products
This is an exciting opportunity to carry out a programme of research supported by Hadley Group, a leading manufacturer of rolled steel products for applications in building sector. Hadley Group have developed the UltraSTEEL dimpling process which won the 2006 and 2014 Queen’s Awards for Enterprise. The PhD will be focused on integrating this process with acoustic enhancements to reduce significantly transmission of sound through lightweight building elements that include Hadley Group steel components. The student will get access to real-word manufacturing processes and data, and expertise at the dedicated Technical Centre of Excellence with over 50 engineers involved in machinery, tooling, product design and R&D activities. The student will also be provided with a substantial financial support to travel extensively in the UK and overseas to visit the manufacturing facilities of the Hadley Group in Europe, Middle East and USA, and present the results of their work at high-profile international events. The student will also receive extensive training in acoustics, machine learning, material science, communication skills, related legislation, commercialisation, marketing and outreach skills.
Specifically, the work will involve computer simulations of sound transmission through lightweight building elements, rollforming UltraSTEEL process, laboratory experiments and site trials of full-scale building elements. A key research challenge is to find a balance between the manufacturability of novel steel products with advanced acoustic performance, material composition, structural integrity, weight and cost.
There will be opportunities to study the use of acoustic metamaterials, advanced vibration damping methods and new types of porous media through computer simulation and experiment. The student will be expected to contribute to the integration of the acoustic modelling capabilities, e.g. based on commercial software COMSOL Multiphysics, with in-house Copra FEA software to achieve the required acoustic enhancement without jeopardising the structural integrity of the building elements and their cost.
This research would be well suited for graduates in mechanical engineering, materials science, manufacturing or other related areas of engineering and engineering design. Experience in acoustics, vibration and numerical simulation with finite element method will be useful. You will be based in the School of Mechanical, Aerospace and Civil Engineering at the University of Sheffield.
Sh.24.3 PhD in Sound Analysis for Predicting Category 1 Ambulance Calls
- Project type: Academic-Led project
- Supervisor: Dr Ning Ma and Professor Jon Barker, University of Sheffield
- Project Partner: Yorkshire Ambulance Service NHS Trust
Your PhD will focus on the development of voice analysis technologies to enhance the prediction and triaging of Category 1 ambulance calls.
Ambulance call centres play a critical role in triaging life-threatening medical emergencies. Category 1 calls, indicating life-threatening injuries or illnesses such as cardiac arrest or severe respiratory distress, demand an immediate response to reduce avoidable fatalities. YAS handles over 1.1 million emergency and urgent calls to 999 annually. experienced call handlers often recognise the severity of cases within the initial 15-20 seconds of a call. However, the accuracy of these assessments can be influenced by factors such as the call handler’s expertise, call volumes, and stress levels, potentially delaying life-saving interventions.
Emerging advancements in artificial intelligence (AI)-driven speech and voice analysis present transformative opportunities to enhance emergency call triaging. For instance, identifying specific audio features, such as laboured breathing or vocal markers of severe distress, could enable earlier and more accurate prediction of Category 1 emergencies. The integration of such tools into call centre workflows promises to improve decision-making speed and accuracy, ultimately saving lives.
This collaborative PhD project aims to develop and evaluate advanced deep learning models for speech and audio analysis to predict Category 1 emergencies, improving the speed and precision of emergency response systems. The objectives include:
- Collaborate with YAS to curate a high-quality dataset of emergency call recordings, annotated with corresponding medical outcomes and severity levels.
- Identify vocal and acoustic biomarkers indicative of life-threatening conditions, including laboured breathing, distressed speech patterns, or cognitive impairment markers.
- Develop machine learning models capable of predicting Category 1 emergencies based on real-time audio features extracted from calls.
- Work iteratively with YAS researchers to test and refine the models, ensuring usability, reliability, and integration into operational workflows.
The successful candidate will benefit from interdisciplinary training in experimental design, advanced speech analysis, and machine learning techniques. Supervision will be provided by experts from the University of Sheffield and industry professionals at YAS. The candidate will also undertake a placement at YAS to gain hands-on experience in real-world emergency call environments, ensuring the practical relevance and impact of the research.
This research aligns with the Positive Uses of Sound theme in the Sound Futures CDT and addresses both national and international health priorities in developing fair and inclusive systems for real-world applications.
Sh.24.4 Better Personalization of Deep Learning-Enhanced Hearing Devices
- Project type: Academic-Led project
- Supervisor: Professor Jon Barker, University of Sheffield and Dr Simone Graetzer, University of Salford
- Project Partner: The Royal National Institute for Deaf People (RNID)
Hearing loss affects over 5% of the world’s population, making it a major public health concern. Hearing aids are the most commonly prescribed treatment, but many users report they do not perform well for listening to speech in noisy situations. Breakthroughs in deep learning and low-power chip design are driving the next generation of hearing devices and wearables, with the potential to revolutionize speech understanding in challenging listening environments. For example, Apple’s AirPods Pro have gained FDA approval as hearing aids for mild to moderate hearing loss, and Phonak has introduced deep neural network-equipped devices that dynamically enhance speech clarity in noisy environments. However, training these approaches to work in general settings and to suit individual preferences remains a critical challenge.
To improve deep learning-enhanced hearing aids, we require metrics that predict how well a given hearing aid algorithm will perform for a specific user in a particular acoustic environment. Existing approaches often rely on oversimplified assumptions about listener preferences, which are captured using basic metrics. For example, it is often assumed there is a well-defined target speaker and that processing should maximise noise suppression while preserving quality. These simple metrics do little to capture users’ needs in more complex settings, such as trying to engage in multiparty conversations in a busy restaurant.
The project will explore a variety of methods to understand hearing device user preferences in more complex settings, including leveraging virtual reality (VR) to simulate diverse acoustic environments and hearing aid algorithms. VR offers the advantage of creating immersive and controlled scenarios where users can directly experience and evaluate different algorithmic configurations. This approach allows the systematic measurement of user preferences across a wide range of conditions, ensuring both ecological validity and experimental rigor. From this understanding new algorithm quality metrics will be derived for optimising existing deep-learning enhancement approaches in a more user-dependent manner.
The project will be based at the University of Sheffield and co-supervised by experts from both Sheffield and the University of Salford, collaborators on the ongoing EPSRC-funded Clarity Project . The Clarity Project focuses on improving speech-in-noise understanding, making it a natural foundation for this work. The Royal National Institute for Deaf People (RNID) will act as a key partner, offering additional expertise and a crucial end-user perspective.